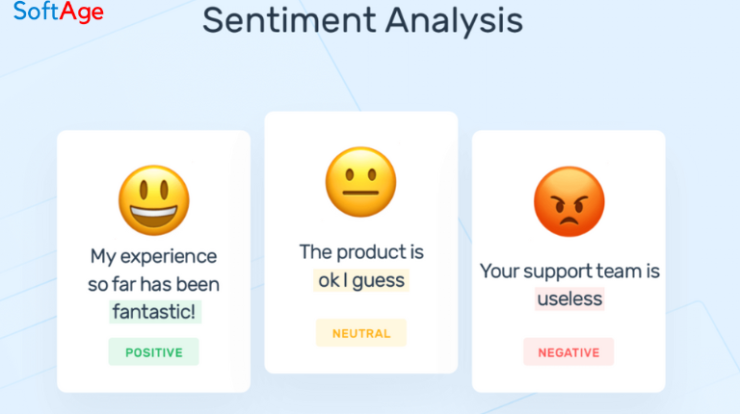
In the digital era, an ocean of text data flows through our screens every day, from social media posts to product reviews and news articles. Understanding the sentiment behind this text can be a formidable task, but thanks to the advent of sentiment analysis, it’s become much more manageable. In this article, we’ll dive deep into the world of sentiment analysis, exploring its significance, methodologies, applications, and the ethical considerations that come with it.
Understanding Sentiment Analysis: What Is It?
Sentiment analysis, often referred to as opinion mining, is a branch of natural language processing (NLP) that focuses on extracting and categorizing opinions, feelings, and emotions expressed in text data. It is a valuable tool for discerning the overall sentiment or emotional tone in a piece of text, ranging from positive and negative to neutral or even more nuanced emotions.
The Significance of Sentiment Analysis:
Sentiment analysis is not just a tool for businesses to gauge customer satisfaction; it holds a wide range of applications across various industries. Here are a few of the key areas where sentiment analysis plays a pivotal role:
1. Business and Market Intelligence: Companies use sentiment analysis to monitor customer feedback, assess product reviews, and gain insights into consumer sentiment. This information can help in product development, brand management, and making informed business decisions.
2. Social Media Monitoring: Social media platforms are rich sources of user-generated content, making them perfect for sentiment analysis. Brands use this data to gauge public perception and engagement, while governments and organizations use it for public opinion monitoring.
3. Customer Support: Sentiment analysis is employed in customer service to quickly assess customer sentiment in emails, chats, and calls. This helps companies route inquiries more efficiently and respond with an appropriate tone.
4. Political Analysis: Sentiment analysis is used in political campaigns and governance to gauge public sentiment and assess the impact of policies and decisions. It is especially relevant in the age of social media, where political discourse is prominent.
5. Content Curation: Media outlets use sentiment analysis to curate content based on audience preferences and engagement, ensuring that the most relevant and engaging content is presented to readers.
Methodologies and Approaches in Sentiment Analysis:
Sentiment analysis can be approached in various ways, depending on the complexity of the task and the available resources. Here are three common methods:
1. Rule-Based Sentiment Analysis: This method relies on predefined rules and dictionaries to assign sentiment scores to words and phrases. For example, the word “happy” might be associated with a positive sentiment, while “angry” might be associated with a negative one. This approach is relatively simple but can be limited by its inability to capture nuances and context.
2. Machine Learning-Based Sentiment Analysis: Machine learning models, including deep learning techniques, are used to build sentiment classifiers. These models are trained on labeled datasets, allowing them to learn patterns and relationships between words and sentiments. They can handle more complex tasks and are capable of recognizing contextual cues.
3. Hybrid Approaches: Some sentiment analysis systems combine rule-based and machine learning-based methods to improve accuracy. These hybrid approaches leverage the strengths of both techniques to achieve a balance between precision and recall.
Ethical Considerations in Sentiment Analysis:
While sentiment analysis offers numerous benefits, it also raises ethical concerns. Here are some key considerations:
1. Privacy: Analyzing sentiment from public posts is generally acceptable, but when dealing with private conversations or data, issues of privacy and consent arise. It’s essential to respect individuals’ privacy and adhere to data protection regulations.
2. Bias and Fairness: Sentiment analysis models can be biased, reflecting the biases present in the training data. This can lead to unfair or discriminatory outcomes, impacting certain groups negatively. Addressing bias in sentiment analysis is crucial to ensure fairness.
3. Context and Nuance: Sentiment analysis may struggle with understanding sarcasm, irony, and cultural nuances. Language is inherently complex, and interpreting sentiment requires an understanding of context.
4. Accountability: Automated sentiment analysis tools can sometimes make incorrect judgments, causing harm or misunderstandings. It’s important to have mechanisms for accountability, including human oversight.
The Future of Sentiment Analysis:
Sentiment analysis continues to evolve as AI and NLP technologies advance. The future of sentiment analysis may include:
1. Multimodal Analysis: Incorporating not just text but also images and audio for a more comprehensive understanding of sentiment.
2. Real-time Analysis: Implementing sentiment analysis in real-time, allowing immediate responses to shifts in public sentiment.
3. Improved Context Awareness: Developing models that better grasp the context and nuances of language, reducing misunderstandings.
4. Enhanced Bias Mitigation: Creating models that are more robust to biases and offer a fairer analysis.
Conclusion:
Sentiment analysis is a powerful tool for unlocking the sentiments and emotions hidden within the vast amount of text data circulating in the digital realm. Its applications are diverse, ranging from business and marketing to politics and media. As the field continues to advance, it is essential to navigate the ethical considerations and challenges it poses to ensure that sentiment analysis remains a valuable and responsible technology in the digital age. Sentiment analysis is more than just a technology; it’s a gateway to understanding the human experience in the digital age, one text at a time.